Our fourth annual State of Payment Operations report is here.Get the Report →
Demystifying AI and LLMs for Finance
This journal clarifies the role of AI and LLMs for finance—specifically, we’ll demystify important concepts and offer suggestions for thoughtful implementation.
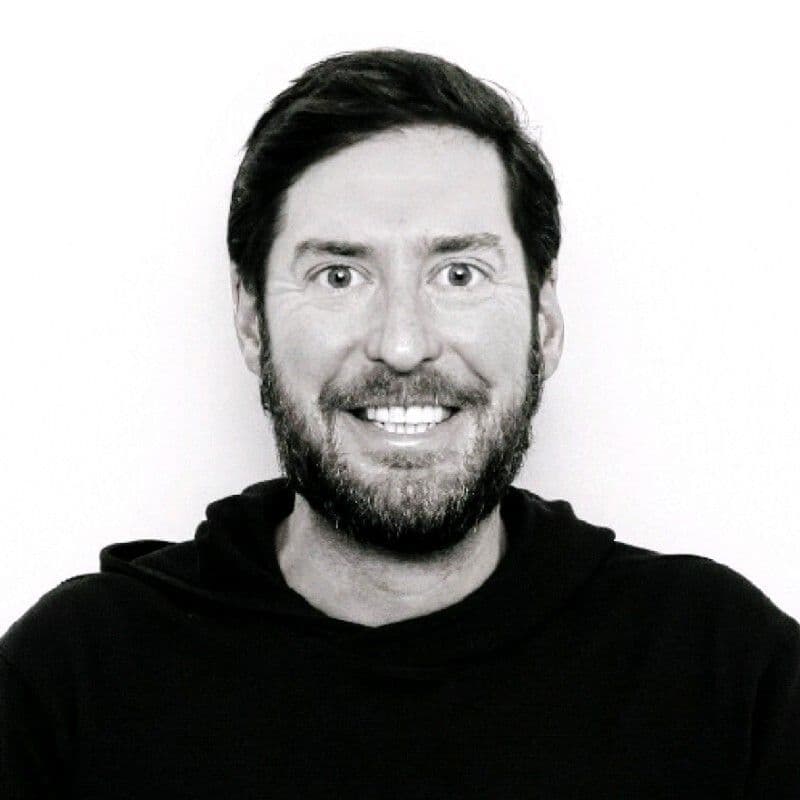
In the last five years, interest in AI on Google has increased by 134%. From Chat GPT’s launch—charting 1 million users within the first five days—to the current landscape around AI and Large Language Models (LLMs), the volume of content and proliferation of buzzwords can be difficult to sift through.
Amidst the hype, at Modern Treasury we’ve distilled our approach to AI into three key principles: customer control, unwavering accuracy, and seamless scalability. With these guiding tenets, our team can deliver AI-based solutions that are as innovative as they are reliable, secure, and impactful for customers.
Five Things Finance Leaders Should Know About AI and LLMs
1. AI is no boogeyman. Trepidation about AI, LLMs, and ML (machine learning) isn’t exclusive to finance. Across professions, technical details regarding how these systems operate can feel elusive. Perhaps the most important thing to know about AI and related workflows is that they are statistical models at heart—not omnipotent robots. AI models suss out nuanced correlations, trends, and concepts from over 20 years of consumer internet data and content, all in a statistical manner. These technologies are best seen as new tools to augment—not usurp—a finance professional’s toolbox, offering more gains to productivity than reasons to fear.
2. ML and Generative AI are not synonymous. While they are both branches of modern AI, ML and Generative AI (GenAI) are not the same thing. What one author called “pattern recognition on steroids,” ML focuses on the analysis of data to make predictions. ML has been in use since before the term was coined by Arthur Samuel of IBM in 1959, with massive use throughout the consumer internet era. GenAI emerged around the same time as ML and expands upon it, performing similar data analyses—namely, GenAI produces outputs in human-readable domains (e.g., text and images). LLMs are a subset of GenAI that use human language for inputs and outputs.
3. The modern AI ecosystem has three parts. The primary components that make up AI in today’s business environment are:
- Foundational models, like OpenAI’s GPT-4, trained on data from the internet
- AI and ML infrastructure, which has been developed extensively over the past 20 years to handle large volumes of data, serving to integrate “value add” AI capabilities into product and businesses
- A vertical application of these tools, determined by how AI meets product development, working back from clear customer pain points.
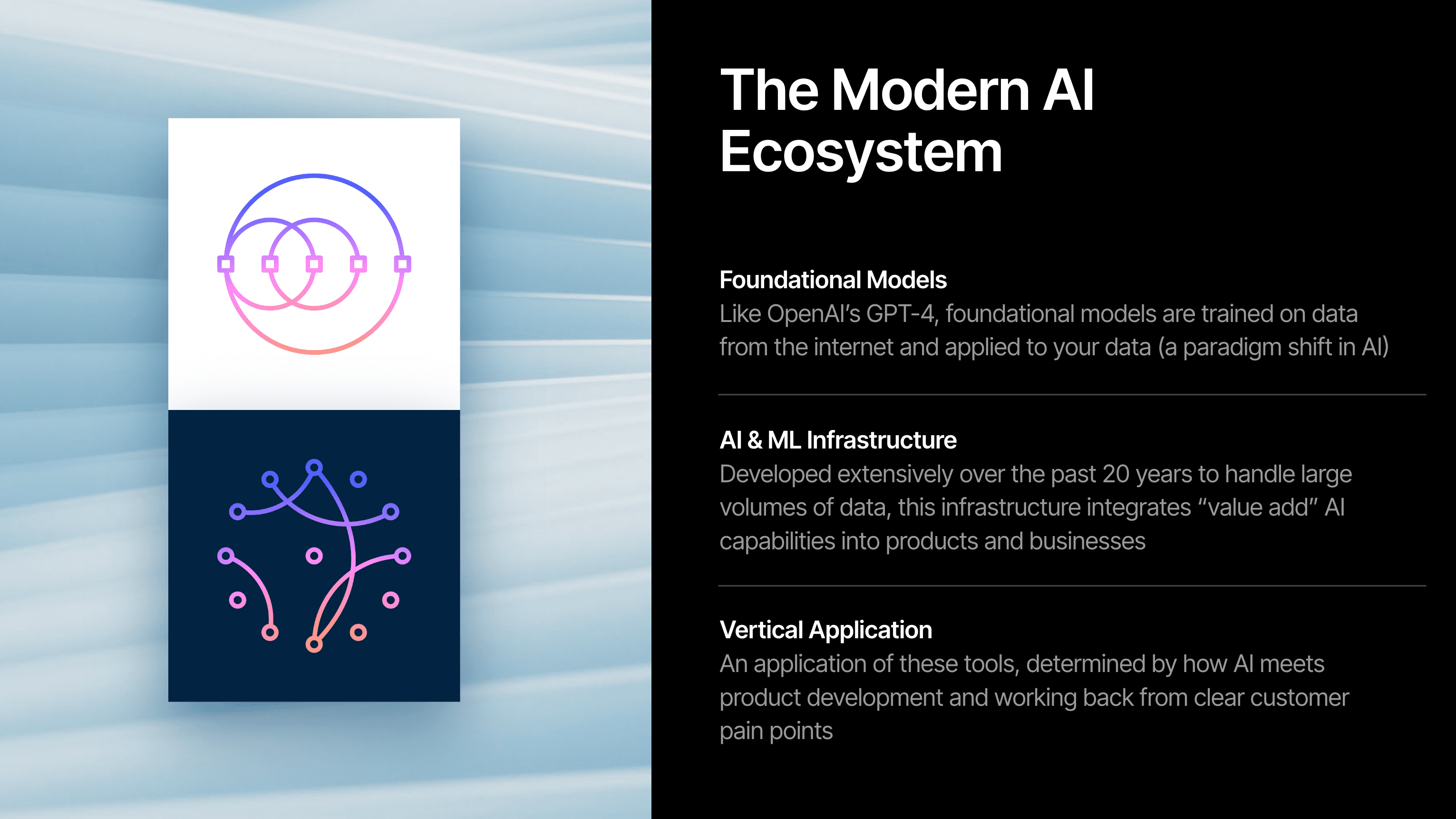
As an example of the latter, suppose a company wishes to apply AI tools to improve fraud detection—specifically, a nuanced financial paradigm for fraud involving text. They might use an LLM as an input in conjunction with other features to train a fraud model that is more accurate than its predecessor. In this case, the company has applied modern AI to a specific domain for a relevant and discrete task.
4. AI-source model choices matter. Modern AI centers around two models: closed- and open-source. Private companies with private LLMs, like OpenAI, use closed-sourced models, wherein users cannot access parameter values or other important details about how a model works. Open-source paradigms, like those employed at Modern Treasury, offer incredible transparency around the particular weights for each model. Users can learn how models were trained, then download models within their own security posture. Companies thus maintain greater control over their most valuable asset (data) by bringing a model to their data versus sending their data to an external model.
5. In a transition period, partnerships count. AI is currently transitioning from the private sector into the public sector. Waves of private and public investment are driving the bulk of resources fueling AI technologies, and the results remain to be seen. If the sea change of the last two years is any indication, more change is afoot. In light of this, finance leaders should ensure they have an expert partner in this domain—one who can provide guidance on resource allocation and investments because there are numerous ways to approach AI and LLMs. Some will bear fruit and some will not.
How AI and LLMS Can Transform Finance
Much like AI itself, finance professionals are responsible for finding answers, making predictions, and guiding decisions based on their synthesis and analysis of abundant data.
Delegating lower-level data-heavy tasks to AI frees up time for:
- Brainstorming and strategy. Free time can be used to consider goals and perform tasks that require critical thinking, such as audit readiness, drafting or reviewing memos, or conducting technical accounting research.
- Balancing responsibilities. It can be a challenge to prioritize operational needs vs. long-term projects. Time is limited—AI can help ensure hours are used in the most efficient and productive way possible.
- Higher-level activities, driven by the big questions. AI might be used like a staff accountant so leaders can focus on inquiries like:
- How can I facilitate the growth of my company?
- How can I push the needle forward on long-term projects?
- How can I personally perform better?
In order to achieve these results—or any significant results with AI—leaders should begin by identifying their most significant pain points and problems to solve. This approach ensures that AI projects align with business goals and produce measurable outcomes. For finance roles, primary challenges tend to center around data. From accounting and transaction categorization to anomaly and fraud detection, finance workflows are often an exercise in reviewing, moving, formatting, and culling information. Data from different systems needs to be connected and linked to create a holistic view of financial standing.
AI can be the ultimate source for better, faster inference samples. AI has the transformative power to process large data sets, automate routine tasks, and introduce new avenues for business growth—all of which can dramatically improve efficiency for finance teams.
Reconciliation: A Model Use Case
Reconciling cash across a high volume of transactions and accounts is a significant challenge for many teams. Enterprises often deal with inconsistent reporting from disparate sources, not to mention routine exceptions and complex matching scenarios. Despite these difficulties, transaction reconciliation is essential for financial control and effective risk management—and AI can make the process less painful.
Certain workflows within transaction reconciliation are particularly well-suited to AI, giving teams time back to focus on exception cases. With every business, data increases over time—for reconciliation, this means more transactions and more financial data to manage. The world of faster payments needs real-time insights beyond bank hours or month-end closes.
Get reconciliation right, from A(I) to Z
From basic concepts to future potential, gain an in-depth understanding of reconciliation to drive your business forward
Modern Treasury incorporates AI in the following ways:
AI-powered data ingestion: Users can easily configure data pipelines for smooth data mapping and normalization. These pipelines can be accessed through CSV uploads for manual tasks and APIs for automated processes.
AI-powered match and rule suggestions: Suggested matching and reconciliation rules enhances reconciliation speed. Recognizing patterns within a company's dataset facilitates faster automation, resulting in better reconciliation rates.
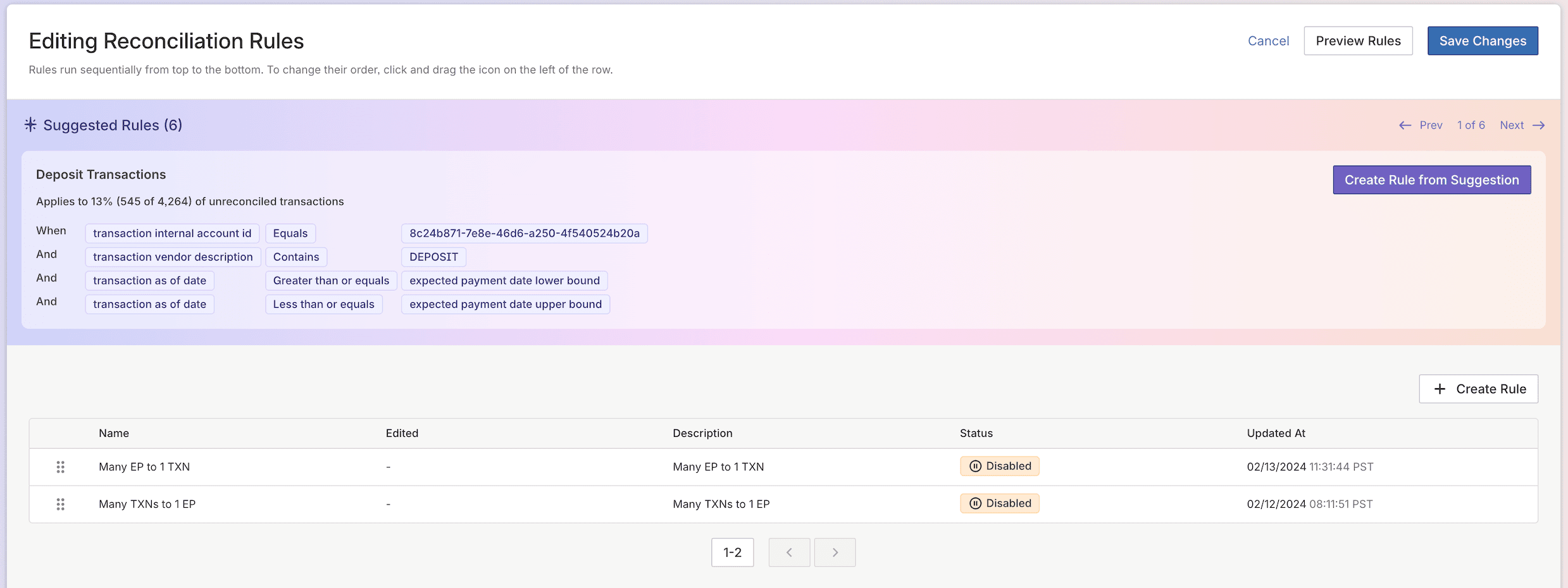
The latter is particularly significant for enterprise finance teams. In the case of a large company with hundreds of reconciliation rules, capturing these incremental patterns becomes increasingly challenging. An AI system that monitors changes in financial data or new fund flows to identify emerging patterns and suggest new rules for a team to review and approve can save considerable time.
Partnering for the Future
Because building foundational AI models can be a steep barrier to entry, many finance leaders will explore purchases and partnerships. It’s important to seek out mature and principled partners who not only work back from clearly defined use cases but also operate through a data governance lens—with respect for where client data resides, recognizing how priceless data is to an enterprise.
The Modern Treasury team has significant experience with financial product development as well as the landscape of finance-related problems for which modern AI, employed in a responsible manner, is transformative. AI and LLMs power our products and leave you in control, helping companies move, track, and reconcile money, at scale and in real time. Reach out today to learn more.
Try Modern Treasury
See how smooth payment operations can be.